Informational analysis to assess diagnostic accuracy of a medical test
Suppose one has a new diagnostic system (a computed tomography or ultrasound scanner, …), or a new diagnostic test for detecting a disease (diabetes, prostate cancer, …). Obviously we need to compare the performance of the new diagnostic system/test with the performance of the old one. How to proceed? It is customary to execute two series of “experiments” (for example 1000 mammography examinations for detecting breast cancer), one with the old method and one with the new one. To compare the quality of the two systems, we need the involvement of the so called “standard of reference” (SR); it is a sort of perfect test, that always gives the correct answer, but that cannot be used in daily practice since it is (for example) too invasive (a painful feeding tube), or destructive (a biopsy), or very expansive, or delayed in time (a follow-up mammogram obtained at last 10 months after). The outcomes of the two tests will be compared to the reports of the SR.
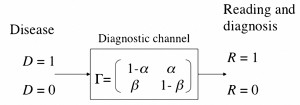
Fig. 1. The model of the “diagnostic channel” underlying informational analysis of a diagnostic test.
In the case of a dichotomous diagnostic test, we have two possible outcomes for it: “yes” (the disease is present,D=1), or “no” (the disease is not present,D=0). But, since the test can make mistakes, it can occur that the test says “yes” when the correct answer would be “no”, or the vice-versa. So, to evaluate a test, it is customary to calculate four numbers, respectively the (percentage of) true positives (orsensitivitySE),假阳性(FP比率),假negatives (FN ratio), and true negatives (orspecificitySP). A test is good when it has high percentage of TPandTN. The SR has 100% for both TP and TN. But how to compare two diagnostic tests, with one having e.g. SE=80% and SP=60%, and the other SE=60% and SP=80%? Unfortunately, up to now, nobody was able to propose a convincing global parameter derived from the above mentioned percentages. Now our innovation.
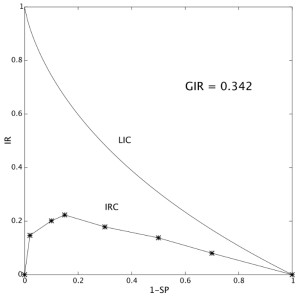
Fig. 2. GIR is the global information ratio, an index calculated by comparing the behavior of the Information Ratio Curve of the test with the Limit Information Curve, that is the best possible diagnostic performance.
A diagnostic test is conceived to gaininformationon the disease of the patient, that is ahiddenobjective status which can be present (D=1) or absent (D=0). The physician makes assumptions on the disease by interpreting the result of the diagnostic test;R=1 means that (it is diagnosed) the disease is present,R=0 means the disease is absent. All this process (e.g., a mammography examination interpreted by a radiologist) can be thought of as the outcome of thediagnostic channelof Fig. 1. So the accurate diagnostic test (or the accurate reader) will be the one able to extract as much information as possible from the diagnostic channel. We know that a perfect method to measure information already exists; it is theMutual Information(MI), which is a mathematical function developed in the framework ofInformation Theory; note that all modern digital communication systems that interact over a network are based (also) on this theory. So we have used MI to compare diagnostic tests, being able to determine the clinical conditions that maximize the flux of information (e.g. with respect to theprevalenceof the disease). We have also introduced an index for the diagnostic test, which cumulates the information among all possibleprevalence scenarios; if we compare it with the same index obtained by the SR, we have the so calledInformation Ratio(IR). This analysis can be easily extended also to tests yielding continuous values (e.g., PSA level) or ordered categories (e.g., 7-point scale in breast imaging), corresponding to multiple cutoffs of specificity. In this case we introduce a curve that illustrates the behavior of IR versus 1-SP (see Fig. 2.) and that is alternative to classical ROC analysis.
Rossano Girometti1, Francesco Fabris2
1Dipartimento di Scienze Mediche e Biologiche, Istituto di Radiologia Diagnostica,
Università degli Studi di Udine, Udine, Italy
2Dipartimento di Matematica e Geoscienze, Università degli Studi di Trieste, Trieste, Italy
Publication
Informational analysis: a Shannon theoretic approach to measure the performance of a diagnostic test.
Girometti R, Fabris F.
Med Biol Eng Comput. 2015 Sep
Leave a Reply
You must belogged into post a comment.