Resting state fMRI analysis using sparse dictionary learning in SPM framework
大脑always be active even people are in rest. In the resting period, it has been observed that particular groups of brain region are always co-activated. These regions are functionally connected each other and each group is called as intrinsic connectivity network (ICNs). They are related with distinct brain functions, for example, visual, auditory, motor, attention, and so on. These ICNs are functionally segregated and integrated according to the brain state and we can estimate brain state inversely using connectivity pattern of ICNs.
There are two well-known analysis method of functional brain connectivity studies, seed-based approaches and independent component analysis (ICA). The seed-based approach extracts information from a specific brain region, called a “seed” region, and computes the similarity between information from the seed and all other brain region to obtain a brain network pattern. Despite their popularity, seed-based correlation analyses have limitations such that they should require a prior determination of the seed’s location. On the other hand, ICA automatically decomposes whole brain network into maximally independent sub-networks based on the independency assumption. However, the brain networks are hardly maintained independently due to their complex interconnection.
In this paper, we presented a novel functional connectivity analysis method that is inspired by the graph theoretical approach. We regard the temporal dynamics at each brain region as a sparse combination of unknown global information flow. In particular, a new concept of a spatially adaptive design matrix has been proposed to represent local connectivity that shares the same information. Unlike ICA methods, the new algorithm is not based on the independency assumption and exploits the fact that temporal dynamics at each voxel can be represented as a sparse combination of global dynamics because of the property of small-worldness of brain networks. If we further assume that local network structures within a group are similar, the estimation problem of global and local dynamics can be solved using “sparse dictionary learning” from compressed sensing theory for the concatenated temporal data across subjects. Moreover, the aforementioned individual and group analyses using sparse dictionary learning can be accurately modeled by a mixed-effect model, which also facilitates a standard SPM-type group-level inference using summary statistics.
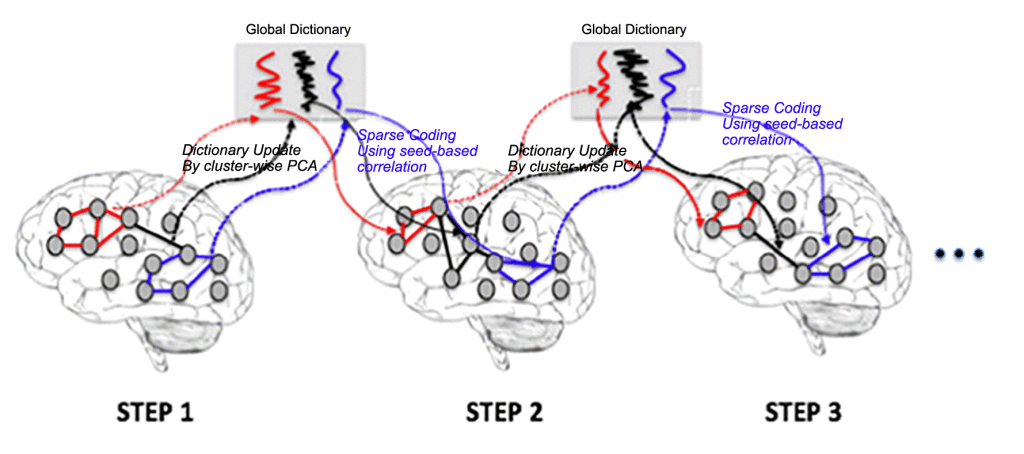
Dictionary update step: For a given set of voxels within a community, the corresponding dictionary atoms are updated as a principal component analysis of the temporal dynamics. Sparse coding step: For each dictionary atom that represents the common temporal dynamics, the most correlated voxels are statistically tested to define their membership for each community.
Among ICNs, A default mode network (DMN) is the most well-known ICN which are highly activated and connected at resting state. Recent studies of functional connectivity have revealed that the DMN activity is disrupted in diseases such as Alzheimer’s disease (AD). Using an extensive resting fMRI data set obtained from normal and Alzheimer’s disease patient groups, we demonstrated that the changes in the DMN extracted by the proposed method are correlated with the progression of Alzheimer’s disease.
We also compared and validated our tools with the existing seed-based and ICA approaches. To compare with ICA methods, our method provided more spatially correlated individual maps compared to the ICA analysis, which is another indication of the robustness of the algorithm. We also showed that our method can extract other subnetworks, which was not feasible using seed-based analysis. Based on the results and unique theoretical advantages, we believe that the results provide strong evidence that the proposed method is a powerful tool for resting-state fMRI analysis.
Yong Jeong
Department of Bio and Brain Engineering
Korea Advanced Institute of Science and Technology (KAIST)
The Republic of Korea
Publication
Sparse SPM: Group Sparse-dictionary learning in SPM framework for resting-state functional connectivity MRI analysis.
Lee YB, Lee J, Tak S, Lee K, Na DL, Seo SW, Jeong Y, Ye JC; Alzheimer’s Disease Neuroimaging Initiative.
Neuroimage. 2016 Jan 15
Leave a Reply
You must belogged into post a comment.